[Resource Post of the Day] How food delivery can be a sustainable business
[Editor's note - The following article was written after going through a medium post on the mathematical analysis of the food delivery companies in India by Dr Santanu Bhattacharya. This article explores the other side of the issue.]
Recently, there have been a lot of articles discussing the feasibility of food delivery startups. Apparently, food delivery business had always been around, but was managed directly by the restaurants in your neighborhood. Restaurants would send across their workers to deliver the food once ready. However, this model had certain issues like only few chose to deliver, delayed delivery during peak hours, not economical for the business to keep full-time delivery boys, dependency on the only worker, very minimal reach, etc. With the growing smartphone and internet penetration came the on-demand food delivery businesses. This model would bring restaurants and customers on a single platform and manage end-to-end logistics, thereby providing convenience to both the parties apart from other facilities like choice, speedy deliveries, availability, etc.
Let’s look at the business model from the ground up to understand its dynamics. The on-demand food delivery business can work only if all the parties involved (namely customer, merchant, aggregator and delivery boy) have enough motivation to be on the platform. Let’s understand the motivations for all four of them:
- Customer: They will stay on the platform if it does these three things better than all the other available options:
o Delivers quality food in promised time
o Reliably serves the request most of the time
o Levies acceptable costs
- Merchant: The motivations are two-fold:
o Getting more customers from the online platform
o Converting the fixed delivery cost into variable cost
- Driver: They would stay on the platform only if they see more earning on the platform as compared to others for the amount of time invested.
- Aggregator: Needs to be financially viable in the long run, while serving the other three parties on the platform efficiently.
It is tough to meet all expectations all the time. Thus, we present a practical solution that can help a company break-even while achieving most of the expectations most of the time. Below are the points we have considered in our business model, which can significantly impact the unit economics and experience of the end consumer.
1) Stockouts: The expectation from any on-demand model is always to serve most of the order requests. The experience loss that happens by delayed delivery of an accepted order is much worse than not accepting the order upfront. This can also be observed with taxi aggregators where a lot of demand is not served due to unavailability of fleet. Hence, like any on-demand model, stockout is a part of this business too.
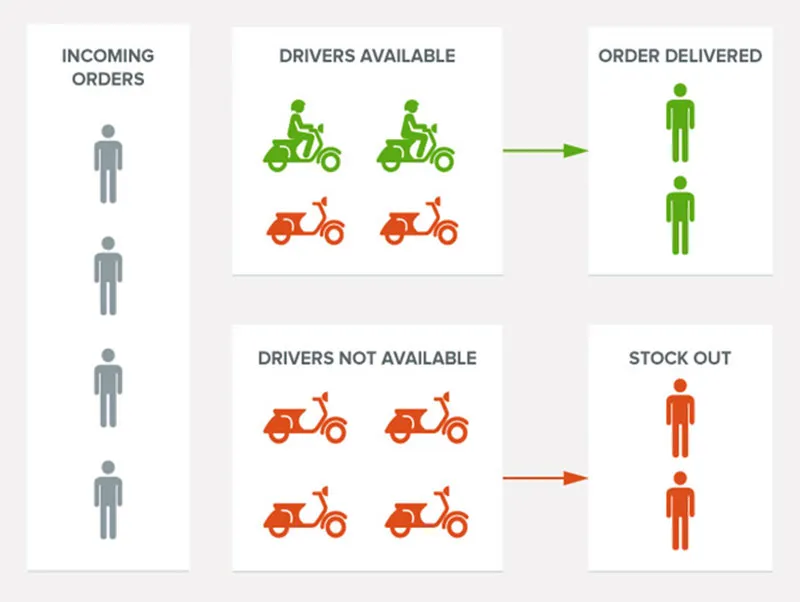
2) Part time drivers: As demand for food is not constant throughout the day, it makes little sense to keep full time drivers. Part time delivery boys are the biggest boon for food delivery/taxis businesses as they help manage peaks and leans economically.
3) Pooling of orders: In an on-demand system, there is always scope for pooling of orders without hurting the ETA. Currently at Runnr, we pool around 10-15 percent of orders, which reduces our service time by three to four minutes. This helps in serving more orders in the system, thereby increasing efficiency.
Stockout calculation using Queuing Model
To model the stockouts mathematically, we used the queuing model. Stockouts are the number of orders which the system will not accept due to unavailability of drivers.
For a customer, a stockout appears as temporary unavailability of certain restaurants in your serviceable restaurant feed.
The Queuing model is a mathematical model that describes how customer’s orders arrive onto a platform and in what manner are they going to be served. Many real world phenomena like queues at railway stations, arrival of customers at a store, etc., can be modeled using queuing theory.
Ideally, we should queue orders that can be served in the promised time. As it is difficult to model that analytically, let’s consider a conservative system, where any order that comes on the platform when no driver is free will be dropped resulting in a stockout. In such a case, our analysis will be based on M/G/m/m [1] queuing model.
To explain it further, M/G/m/m model can be characterised by four parameters:
- Order arrival distribution (M) - Order arrival process is assumed to be a Markov process where the number of new arriving customers is completely independent of the arrival of last customer on the platform. One of the prominent ways to model a continuous time Markov process is Poisson process [3]. Parameter for Poisson process is the arrival rate (lambda), which is nothing but order arrival rate in our case.
- Service time distribution (G)- This is the distribution of the amount of time spent on one delivery. From our data, we found our service time distribution to be gamma distribution with a mean of 30 minutes. Also, around 10 percent of orders are clubbed in a bundle of two, which further decrease service time to 27.3 minutes in our case.
The following graph shows the distribution of service time, which resembles Gamma distribution.
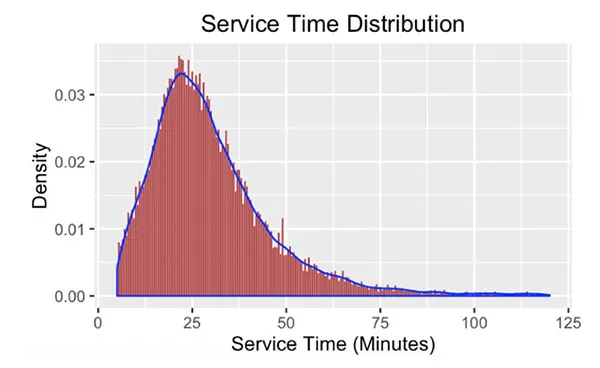
- Number of delivery boys (m): ‘m’ is the number of delivery boys present on the platform. It is analogous to the number of ticket counters in a railway station.
- Number of customers in the system (m): In this model, the maximum number of customers on the platform at any given time is taken to be same as the number of delivery boys. Hence, there will be no customers waiting in queue on the platform. In reality, our system uses smart queuing, wherein only those orders are queued for which we can ensure that waiting time is not greater than the promised time.
How is stockout calculated?
In our model, stockout will happen at the time when all the drivers are busy. This is same as the blocking probability for M/G/m/m queuing model given by following formula.
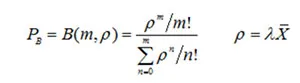
Where, PB= Blocking probability/stockout
m= Number of drivers
λ= Mean arrival rate per hour
x̅ = Mean waiting time per hour
Using the above formula, stockouts for different arrival rates are calculated keeping the waiting time equal to 30 minutes. Based on calculations we made, stockout seems to be changing linearly with increase in arrival rate when the number of drivers are kept constant.
The table below presents stockout percent for fixed number of drivers and varying order arrival rates.
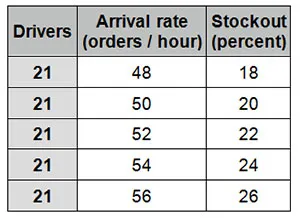
The following graph shows linear increasing stockout with an increase in mean arrival rate.
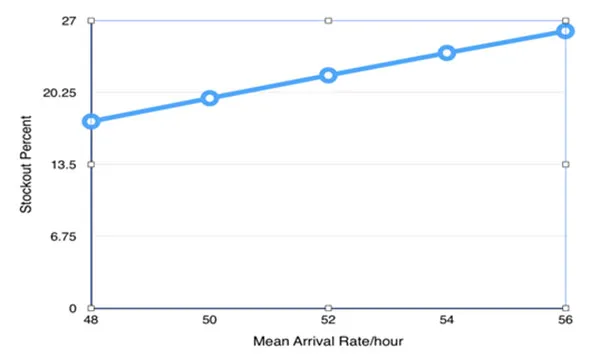
Too geeky? Let’s talk about money
There has been a lot of buzz and conversations at various platform regarding the long-term sustenance of this business model. However, our analysis shows that profitability can be obtained at scale (approximately 100,000 orders a day).
One of the blessings of an on-demand model is that it is highly beneficial for people looking to work part time. Their pay is directly proportional to the amount of work they do and not the number of hours they are logged in to the platform.
For analysis sake (to be on the conservative side), let's consider that they are paid hourly. For cities with a high cost of living like Bengaluru and Mumbai, a delivery boy’s salary is Rs 18,000 per month for working eight hours/day and 26 days/month. This amounts to an hourly rate of Rs 86, which is a cost in the system. Apart from the delivery boys’ salary, there are some fixed corporate overhead costs that are also included in the analysis. From a revenue standpoint, let’s assume that we charge Rs 50 per order as the delivery fee to the merchant.
Using the above cost and revenue structure, the table below lists the number of drivers needed on the platform to break even at different arrival rates. We can observe that we can break even over a large range of arrival rates. This clearly proves that even with conservative revenue and cost structure, it is possible to break even.
At Runnr, during peak hours we have an average and maximum order arrival rate as 42 and 54, respectively.
The table below presents the minimum stockout we need to accept for breaking even at different arrival rates. These stockout numbers are pretty acceptable as per industry standards since taxi aggregators have much higher stockouts.
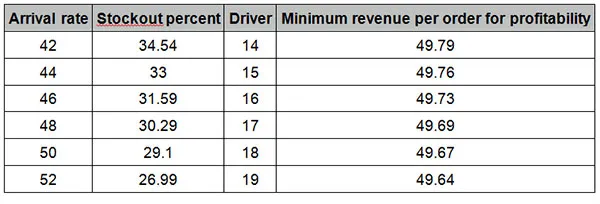
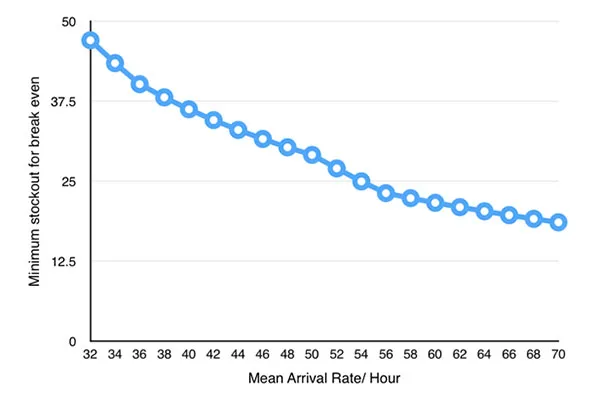
Another observation from the above graph is that as the arrival rate goes higher (increase in demand), we are able to break even with improving customer experience (reduction in stockout).
Conclusion: It ain’t over till it’s actually over!
From the above analysis, it is quite evident that the food-tech businesses have ample opportunity for startups if they stick to correct modus operandi. The online food ordering sector in India has a very high growth rate (more than 30 percent [2]). Keeping in mind the fact that offline food ordering is still dominating the food ordering sector, food-tech businesses will grow at an exceptional rate with increase in the penetration of internet and technology among the Indian middle class.
Apart from the scope of scaling, this sector offers a lot of avenues for optimisation. The whole business model can be made more efficient and profitable with the help of various optimisations such as:
- Commission from merchants: As the food business is shifting from traditional offline mode to online mode, merchants see an obvious benefit of exploring additional channels for demand generation. As a result, they are willing to share a part of their profit as commission, which stands at 14 percent of the order value currently on the Runnr platform. This can further be validated from our internal surveys which show a clear shift over the past one year in market acceptable rates for commission as well as average order value.
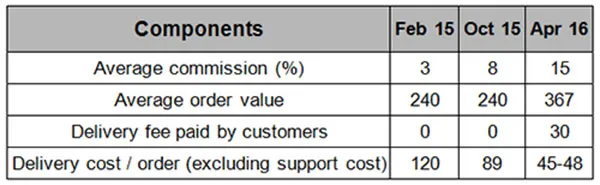
- Smart queuing: In our analysis, we have not considered queuing of any sort. However, a fraction of the orders can be queued if we ensure that waiting time doesn’t shoot up above promised time. Smart queuing can further help in reducing stockouts in the system.
- Delayed dispatch: Delayed dispatch is a concept wherein the dispatch of a delivery boy is delayed so that he doesn’t spend time at the merchant’s location waiting for food to be prepared. Currently, for every order, a delivery boy spends around five minutes waiting for food to be prepared at the merchant’s location. With some advanced data science, we have developed a model to estimate the food preparation time of each merchant. We are in the process of using this estimate to delay the dispatch of delivery boys, thus saving few crucial minutes from service time. Cutting down on service times increases efficiency, thereby reducing the cost.
- Surge: Surge is a big need for an on-demand model as it can convert a significant part of your stockout into high revenue transactions. In simple terms, it reduces stockout by increasing supply as well as decreasing demand.
- Delivery executive payout: In our analysis, we have assumed that delivery boys are paid a fixed amount per hour they login. At Runnr, drivers are always paid based on the number of touchpoints they serve. A touchpoint is defined as any destination where the driver takes some action, for example, for a usual order, there are two touch points (one at the merchant and the other at the customer). This prompts higher logins during peak hours, where there is a high chance of getting an order as compared to lean hours.
From above analysis it is evident that full stack food delivery is a sustainable business. Although there are multiple ways to achieve it, this represents one of them.
References
- National Programme on Technology Enhanced Learning website- http://nptel.ac.in/courses/117103017/26
- Internet and Mobile Association of India-http://iamai.in/media/details/3692
- Athanasios Papoulis and S.Unnikrishna Pillai, Probability, Random Variables and Stochastic Processes, 4th edition, McGraw Hill, 2002
About the Authors:
Ankit Jain: Ankit works as a Senior Data Scientist at Runnr, where he uses advanced machine learning and programming skills to solve the complex last mile logistics problems in India. Prior to this, he has worked in variety of data science roles in Silicon Valley at Facebook, Bank of America and Clearslide. He holds a master’s from UC Berkeley and bachelors in Electrical Engineering from IIT Bombay.
Amol Sahasrabuddhe: Amol works as a Senior Data Scientist at Runnr. Prior to that, Amol has worked as a quant at Deutsche Bank in algorithmic trading. Amol holds a master’s degree from RPI New York and bachelors in Electrical Engineering from IIT Bombay.
Ashutosh Ranjan - Ashutosh is working as Data Science Intern. at Runnr. He is currently fifth year student at E & EC department, IIT kharagpur. He has also done internship at Quantiphi Analytics.
(Disclaimer: The views and opinions expressed in this article are those of the author and do not necessarily reflect the views of YourStory.)