Here is why you shouldn’t trust statistics
Statistics play a huge role in our lives. We not only use them for academic purposes but also for real life activities. While on one hand, something as simple as choosing a certain product over another is determined by some statistical data presented to us, on the other hand, bigger matters like some innovation, medical breakthrough etc. is also dependent on statistics.
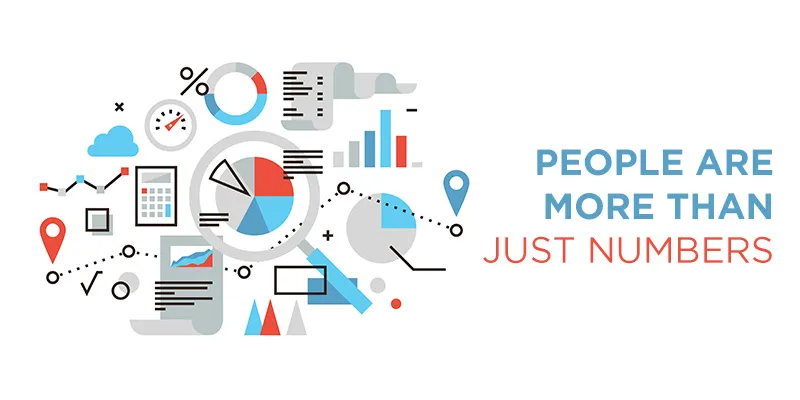
Image : shuterstock
It is often said that misrepresented information isn’t necessarily a lie but a half-truth. Misrepresented statistics can, hence, be called as half-truths. Charles Seife, an American author and journalist, elaborates on this idea in his book Proofiness: The Dark Arts of Mathematical Deception. He states that the pervasive effect of misleading statistical data doesn’t just affect on a micro basis but on a macro basis as well. He believes that it can be used:
“to bring down beloved government officials and to appoint undeserving ones (both Democratic and Republican), to convict the innocent and acquit the guilty, to ruin our economy, and to fix the outcomes of future elections.”
It would make sense to delve further into the topic and understand how statistics can go wrong. Here are two simple ways how this can happen:
Unfair sampling
In the 1976 US Elections, where Jimmy Carter and Ronald Raegan were the two main Presidential candidates, the media came up with innumerable statistical data. One noteworthy case was the poll conducted as a part of the Republican campaign against Jimmy Carter in his hometown. They randomly picked five people from Georgia and all of them had something bad to say about him. When the statistics were presented, it was only natural to presume that Carter was extremely unpopular in his own hometown. However, that wasn’t the truth – although Carter lost the election, he did win the polls in Georgia.
The problem with such a sampling is best explained with this simple example: If a sample is small, the sample will have very different properties from the overall group. If we pick four people at random, one out of sixteen times, they will be all women and one out of sixteen times, they will be all men. Both cases would fail to give a realistic picture of people as a whole.
With the variation in quality and quantity for the same study, the outcome of the statistical data can be completely different. Hence, the randomness of a sample is the reason why such statistical data cannot be trusted blindly.
Statistics as ranks and percentages
Often the person responsible for presenting statistical data as news would want to create a dramatic effect. As a result, instead of showing absolute values, they put forth the results as percentages or ranks.
For example, if a certain startup had to opt for drastic cost-cutting due to unavoidable circumstances and then chose to lay off the employees, the way the statistics is presented in this case actually makes a lot of difference. Let us assume that the startup had only 40 employees and it has to lay off 4 people. This is a small number and doesn’t have any dramatic effect. To beat this, the news will phrase it as 10 percent of the employees have been laid off. Now that certainly has a more shocking ring to it!
Similarly, saying that a certain startup is the best in the city doesn’t make any difference. In fact, for all you know, that company might be making less business. The problem with rankings is that the comparisons are often unclear. You may not know what has been compared with the value in question.
Hence, it is advisable to take decisions on your own without being driven by data from unreliable sources.