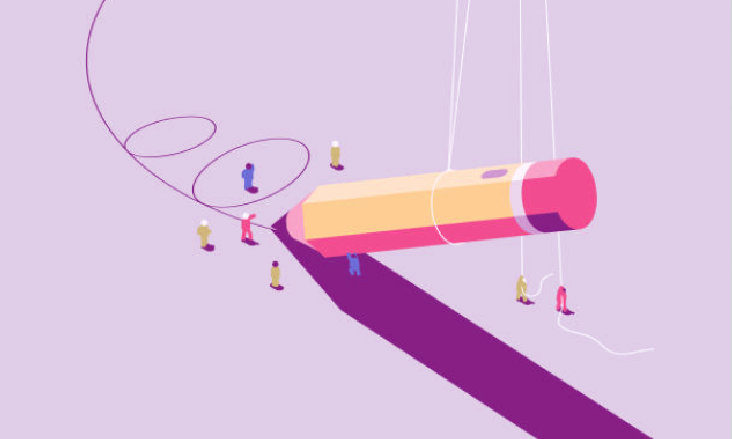
Fraud Detection Improves By Using Artificial Intelligence
Online financial transactions are on the rise. What is the need of AI in Fraud Detection/Prevention? Read on to know more.
Online financial transactions are on the rise. So is fraud. Fraud detection after the event means one has to engage in tracing the culprit and possibly try to recover defrauded amount. Being able to pinpoint suspicious activity that could possibly point to attempts at fraud and thus prevent one from taking place is much better option. This is where artificial intelligence coupled with machine learning, its subset, leads to huge improvement.
The need for AI in fraud detection/prevention
Business suffers increasing losses as fraudsters refine their tactics and strategy one of which is using big data. Sophisticated fraudsters make use of machine learning to detect vulnerabilities in the network chain and big data analytics in real time. Fraudsters attack the weakest and most vulnerable link. Fraudsters work on ways to conceal or shift identity to overcome attempts at detection. They may work in rings and create confusion. They may resort to the simple expedient of mimicking good customer behaviour and thus fool even the smartest automated fraud prevention or detection technology. One technique employed in retail is to take over the account and hack user name and password instead of just the transactions. Valid user name and password can fool the system and fraudsters can steal. This is outright challenging for the human mind and even machine learning and AI can stumble when they come across such tactics. In such cases AI delivers data that human minds can interpret and humans feed data that machines can learn from.
How it works
Fraud detection and prevention become easier through machine learning. Huge amounts of data can be fed to capable machines that can analyze suspicious behavior against past records and flag such activity. Instead of relying on humans, the burden of detection and prevention is passed to machines that analyze streaming data and signal patterns that could point to fraud. Machine learning involves machines that learn from huge amounts of data and have the capability to become more refined over time. However, the flip side to this is that machines can also come up with false positives that could harm genuine customers and genuine transactions.
The answer to this is refined anomaly detection in supervised and unsupervised models. It takes time to implement supervised models in which machines receive training on tagged transactions. It takes massive data for the machine to approach high levels of accuracy. Machines can also be programmed to self learn in the unsupervised model of artificial intelligence. Transactions that do not conform to a set pattern are identified. Behavior pattern sets integrate transaction profiles, behavior sorted lists that identify activities unique to each individual, merchant profiles and self calibrating profiles. Machines also need to be able to distinguish between special and generic behaviors. Then there is a point at which a normal customer may depart from his normal behavior and it could be interpreted as an attempt at fraud. Can the system distinguish such activity? Adaptive analytics could lead to more precision.
AI in fraud is dependent on machine learning that, in turn, is dependent on humans who feed it data it can learn from and the underlying neural networks as well as other algorithms. Today, hardware is up to the task to analyze large amounts of streaming big data in real time and enable decisions with low latency. Accuracy increases as machines self learn and detecting anomalies can be easy. How efficiently fraud prevention or detection is performed depends on the algorithms in use.
Algorithms
Various algorithms are in use in the background to power machine learning designed for fraud detection and prevention. The decision trees algorithm requires less tuning, generates patterns and has good predictive powers. Deep learning enables multiple levels of representation and it has good predictive powers. Similarly, support vector machines have good predictive powers and ability to detect complex patterns. Likewise, neural networks possess predictive powers and ability to analyze complex patterns. Each one has limitations too. It is up to the developer who implements AI based fraud prevention and detection technology to choose the right set. In any case it takes time to develop and implement since machines must get voluminous data to analyze and learn from. Specific data that defines fraud is not available in large number so that makes the task for machines to learn a bit more difficult. The result is that genuine transactions may be blocked much to the annoyance of customers and loss of reputation for the business.
Even if one considers that AI and machine learning are in their infancy, implementations of these technologies have resulted in reduced incidents of fraud. AI will evolve even more in the decade to come and promised to bring greater security and refinements in fraud prevention and detection.